Simulation Modeling Validates Material Handling System Design
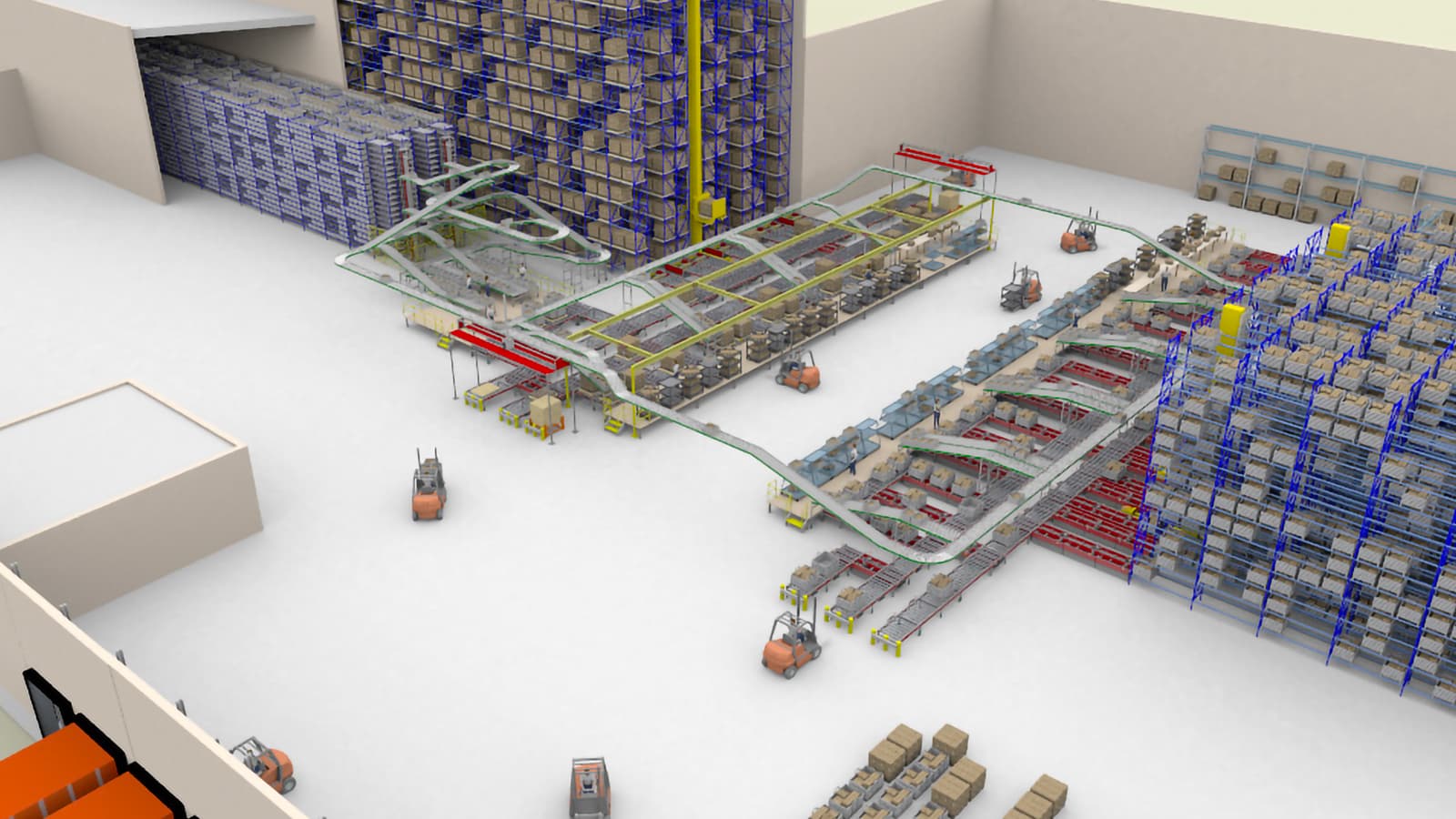
Computer simulation is abstract modeling that uses mathematics to simulate an actual system. Dematic experts use computer simulation to explore and gain new insights into the engineering design of a project. This helps us to estimate the performance of complex systems before they are built.
You wouldn’t think of buying a car without first taking it on a test drive; so why implement a new manufacturing logistics system without first testing it? With computer simulation modeling software you can do just that: test drive your material handling system configuration before finalizing the system design.
When test driving a car, you want to select a route that best evaluates the car performance. Similarly, simulation models can be constructed to include the routes and operational attributes that support the objectives of the design analysis.
Simulation modeling is a critical part of the system design engineering phase. A detailed simulation model is developed based on layout and operating parameters. PLC logic is simulated along with the logistics software.
Material flow rates used in these models are derived from the forecasted production rates. Typical inputs include user data reflecting material flow details from normal to peak operating days.
Simulation modeling determines the operating time required to effectively process low, normal, and peak throughput. These models refine the engineered system design, staffing levels, PLC control logic, and software functionality.
If simulation engineers discover performance issues, the design engineers can develop alternative layout and control configurations.
The proposed revisions to the system are tested with the simulation model. The use of a simulation model helps ensure that the material handling equipment, controls, and software will be capable of handling all the expected operating scenarios.
The impact of the ebb and flow of throughput was assessed successfully. Issues were resolved before the system was actually implemented. Simulation models can evaluate operator performance at workstations. Recommended changes to the system design were tested and evaluated.
Simulation case study
A durable goods manufacturer wanted to evaluate the performance of their proposed material handling system design. Given the size and scope of the project, simulation was employed from the start. The model acted as a focal point for understanding the performance of the material handling system and evaluating alternate configuration options.
The layout included kitting workstations, a work-in-process buffer using an automated storage/retrieval system (ASRS), intra-plant delivery using an automatic guided vehicle system (AGVS), and conveyor.
Containers moved in a sequence between the workstations and buffer storage. The model was used to validate:
- Work-in-process buffer capacity
- AGV fleet size
- Work assignment software algorithms.
The labor needed in each functional area was determined along with the shift staggering pattern. Sensitivity analysis on the conveyor and buffer storage sub-systems revealed potential operational bottlenecks.
Simulation modeling helped to identify system layout and operational changes to the design. Experiments conducted using the simulation model generated several tests for system layout changes, modified flow paths, and revisions to work flow scheduling algorithms.
The model developed included precise operational details of the kitting staff, fork truck drivers, and other relevant production floor associates. Actual production profiles were used to drive the model. The simulation model included the load movement and storage tracking functionality of the software.
The use of a simulation model helps ensure that the material handling equipment, controls, and software will be capable of handling all the expected operating scenarios.
Benefits of simulation modeling
Simulation modeling captured the complex interactions. Because the scope included manufacturing production data, work-in-process buffer size, load sequencing, intra-plant delivery, and kitting operations, the predicted system behavior results were very accurate.
The simulation model included detailed labor and production capacity data. As a result, the simulation model was able to estimate the staff size required to operate the system. In these models, staffing was a variable and performance as a function of staff size could be tested.
The impact of the ebb and flow of throughput was assessed successfully. Issues were resolved before the system was actually implemented. Recommended changes to the system design were tested and evaluated.